views
Introduction
Artificial intelligence is revolutionizing the automotive industry at an unprecedented pace, enabling safer, smarter, and more efficient vehicles. But behind every self-driving car, predictive maintenance system, or AI-powered navigation feature lies one crucial element: data. Specifically, the quality and breadth of data collection for automotive AI.
This blog post explores the importance of high-quality data collection, cutting-edge methods, and how the human-in-the-loop approach transforms raw data into actionable insights for creating advanced AI/ML models. Whether you're an AI engineer, data scientist, or a stakeholder in the automotive industry, keep reading to uncover how data drives safety, innovation, and the autonomous vehicles of tomorrow.
Why Data Quality is Critical for Automotive AI
Data serves as the backbone of all AI applications in the automotive sector. Autonomous vehicles, in particular, require massive amounts of labeled and verified data to make real-time decisions that ensure passenger safety and efficiency. Without high-quality data, even the most sophisticated AI models falter.
The Impact of Poor-Quality Data
Poor data quality significantly impacts the performance of AI/ML models. For instance:
- Erroneous Predictions: AI systems trained on noisy or inaccurate data are prone to false positives and negatives, leading to critical safety hazards in self-driving vehicles.
- Algorithm Bias: Biased data creates systemic errors, like vehicles failing to identify certain objects such as pedestrians.
- Operational Inefficiency: Poor-quality data reduces model efficiency, resulting in higher fuel consumption, decreased route optimization, and lower customer satisfaction.
At Macgence, we specialize in collecting and validating high-quality data. By using advanced data collection techniques and stringent validation processes, we ensure they meet the highest standards for AI model training.
Why High-Quality Data Wins
AI requires structured and consistent data from a variety of input types, including sensors, cameras, and LiDAR. High-quality datasets enable:
- Improved Decision-Making: AI models can make faster and accurate decisions in real-world traffic situations.
- Higher Safety Standards: Reliable data ensures smoother interactions between autonomous vehicles and their surrounding environment, whether it’s maneuvers on highways or crowded urban streets.
- Enhanced Customer Experience: Accurate predictions and personalization foster trust among users.
How Human-in-the-Loop Improves Data Quality
Automation plays a crucial role in data processing for automotive AI, yet humans remain an indispensable part of the process. Enter the human-in-the-loop (HITL) approach, a paradigm that blends automated systems with human expertise to enhance data accuracy and relevance.
What is Human-in-the-Loop (HITL)?
HITL integrates human judgment into the data labeling and training cycle. While automated systems handle large-scale processing, human experts review, validate, and refine outputs to ensure nuanced understanding and quality control.
Benefits of Human-in-the-Loop
- Contextual Understanding:
Humans can interpret subtle contextual cues that machines may not fully grasp, such as distinguishing between objects that appear similar under certain lighting conditions.
- Error Correction:
Automated data annotation systems can mislabel items. Human reviewers ensure errors are caught and corrected before the data reaches AI training pipelines.
- Training Complex Scenarios:
From roundabouts to multi-lane highways, human reviewers help ensure rare and complex driving scenarios are accurately reflected in datasets.
At Macgence, the human-in-the-loop method allows us to deliver precise, reliable data to train robust AI/ML models for autonomous systems.
Proven Data Collection Methods for Automotive AI
The efficiency and reliability of automotive AI hinge on the methods used to collect data. Here are the key data collection approaches we rely on at Macgence:
1. Sensor Data Collection
Sensors from vehicles (e.g., LiDAR, radar, ultrasonic sensors) generate real-time data about surrounding environments. This raw data captures vital details such as vehicle speed, distance from obstacles, and road conditions.
2. Camera-Based Visual Data
Computer vision models rely heavily on extensive visual datasets. Cameras attached to vehicles record everything from lane markings to traffic signs, creating a rich visual database for AI systems.
3. Environmental Data
AI must adapt to changing conditions like snow, fog, and heavy rain. Environmental data drawn from weather sensors equips the AI with the ability to handle challenging scenarios effectively.
4. Annotation for Scalability
High-quality annotation transforms raw datasets into actionable insights. Annotations for traffic signs, pedestrians, and vehicle positions prepare AI systems to make informed decisions.
5. Crowd-Sourced Data
Driver behavior and vehicle diagnostics from ride-share fleets provide scalable, real-world data that reflects diverse traffic scenarios and regional driving patterns.
By employing these methods, Macgence ensures AI systems receive diverse and validated datasets that reflect the complexities of real-world driving environments.
Data-Driven Safety and Innovation in Automotive AI
Safety is a critical driver behind the development of automotive AI. With high-quality data from diverse inputs, innovation takes center stage.
Driving Safety Through Data
AI-powered vehicles can now:
- Detect objects like pedestrians, cyclists, and other vehicles with pinpoint accuracy.
- Predict and respond to sudden traffic pattern changes, avoiding potential accidents.
- Communicate with other vehicles and infrastructure to reduce collision risk.
Pioneering Innovations
Data collection fuels innovation in:
- Predictive Maintenance:
By analyzing vehicle sensor data, AI predicts part failures or maintenance needs, reducing downtime.
- Customized Services:
Personalization enabled by AI enhances the driver’s experience, adjusting settings such as climate controls and infotainment options.
- Smart City Integration:
Integration with smart infrastructure promotes smoother mobility through real-time data sharing between vehicles, traffic systems, and pedestrians.
How Macgence Leads the Way in Automotive AI Data
At Macgence, we empower AI engineers and data scientists with the most comprehensive and accurate data collection for automotive AI. By integrating a human-in-the-loop approach with cutting-edge technology, we bridge the gap between your AI goals and operational reality. Our commitment to quality and innovation ensures vehicles trained with our datasets set new benchmarks in safety, efficiency, and reliability.
Are you ready to revolutionize your AI systems? Contact us today to access world-class data collection services tailored to your needs.
Transforming Automotive AI with Data
Data quality, collection methods, and human expertise are at the heart of advancing automotive AI. By leveraging technologies like sensor data, crowd-sourced data, and the human-in-the-loop approach, innovative enterprises like Macgence are driving the automotive industry into a safer, smarter future.
If your goal is to build AI models that redefine autonomous driving, start with better data. Choose Macgence for unparalleled support in data collection, validation, and AI training.
Contact us today to learn more!
FAQs
1. Why is data quality so important for autonomous driving AI?
High-quality data ensures accuracy in AI predictions, which is critical for safe and efficient vehicle operation. Errors in datasets can lead to incorrect decisions, compromising safety.
2. How does Macgence collect data for automotive AI?
Macgence uses a mix of sensors, cameras, and environmental data collection, enhanced with a human-in-the-loop approach to ensure accuracy and relevance for AI training.
3. Can AI be fully reliable without human-in-the-loop?
While automation handles large-scale processing efficiently, human-in-the-loop adds critical expertise to interpret, validate, and refine the data for optimal performance. Removing human involvement may compromise adaptation in uncommon scenarios.
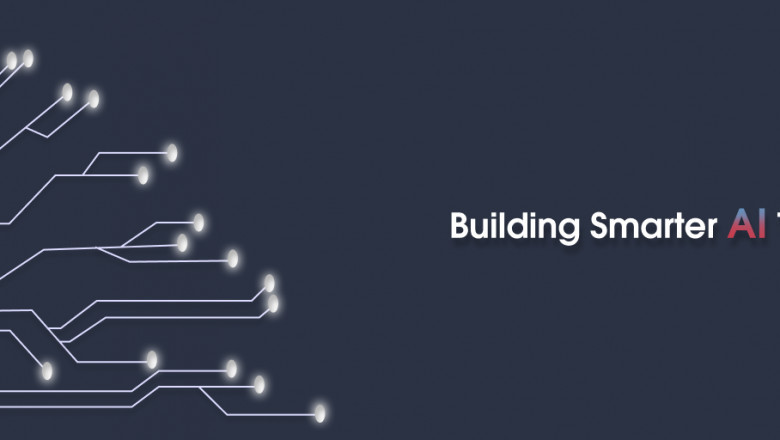

Comments
0 comment